OpenAI is a significant player in the modern artificial intelligence (AI) and machine learning (ML) space. While the parent company is a non-profit, it also consists of a for-profit company that received a $1 billion investment from Microsoft in 2019.
OpenAI has become well-known for its novel and innovative AIs, including Jukebox, a neural net that generates music, DALL-E that generates images from text, and GPT-3, which produces human-like text. Amongst their projects is CLIP, which is designed to learn visual concepts from natural language. CLIP uses image-text pairs available publicly on the internet.
To pre-train CLIP, CLIP is introduced to 32,768 randomly sampled text snippets to discover which one is correctly matched with a specific image. CLIP then replicates this on new images to match visual concepts with language. The result is a highly effective AI that has demonstrated its accuracy with several complex image classification tasks.
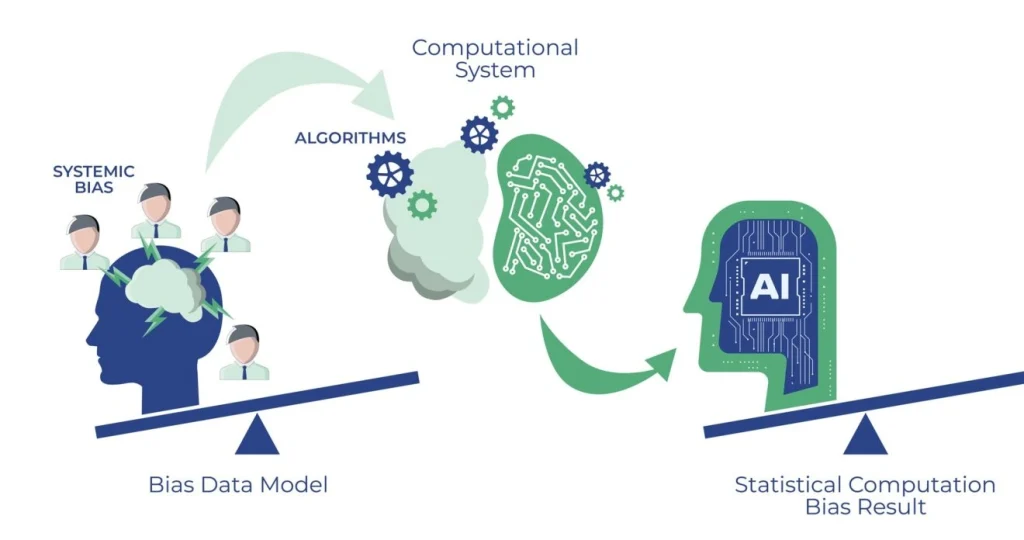
In contrast to the at-times clandestine inner workings of top AI companies, OpenAI lives up to its namesake by openly auditing its research. In 2021, OpenAI released an ‘open audit,’ which confirmed that CLIP was subject to bias. They found that CLIP’s supervised learning process resulted in the AI embodying the biases contained in the internet content it learns from.
CLIP’s designers write: “CLIP attached some labels that described high status occupations disproportionately often to men such as ‘executive’ and ‘doctor’. This is similar to the biases found in Google Cloud Vision (GCV) and points to historical gendered differences”. Objectively speaking, the gender gap in healthcare especially is narrowing. For example, in the UK, women recently outnumbered men in some medical disciplines, such as psychiatry, and globally, women tend to outnumber men in immunology, genetics, immunology, dermatology, and obstetrics and gynaecology.
One of CLIP’s touted benefits was that it eliminates the need for costly image classification datasets; “CLIP learns from text–image pairs that are already publicly available on the internet”, but it appears that using the internet as a data source doesn’t produce ideal outcomes either.
Giving AI free reign to learn from public internet data might seem to answer the issue of training AI with a high volume of data, but the limitations have been exposed.
CLIP Perpetuates Racist and Sexist Bias And Prejudice
In 2022, a team of researchers at the Georgia Institute of Technology in Atlanta set up a new experiment that saw CLIP match passport-style photos with text prompts.
The team set up the experiment in a computer-generated environment – CLIP was given ‘blocks’ that contained people from different genders and ethnicities and was asked to place them into a ‘box’ that described them.
This is the kind of task in which CLIP should excel, as an AI capable of predicting niche images even at low resolutions.
The results of the experiment confirmed that CLIP’s decisions embody the biases contained within its internet-based public dataset. For example, black men were 10% more likely to be associated as criminals, and black and Latino women were more likely to be categorized as “homemakers.”
Clearly, deploying such a model into real-life scenarios is problematic.
Bias Is Not a Theoretical Matter
It’s straightforward to understand why CLIP and other AIs that draw solely from internet data sources are biased – the internet data it uses as a knowledge base is biased.
The internet is phenomenally massive – the largest information system on the planet. But, it’s a relatively modern creation and fails to embody humanity’s longitudinal cross-cultural knowledge.
Additionally, the internet is not equitable – some 3 billion people have never even accessed the internet, let alone contributed to it.
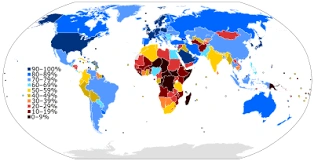
Moreover, some 60% of content on the internet is written in English, despite only around 25% of its users speaking English. Therefore, bias doesn’t just arise from the demographics of internet contributors; it arises from how concepts are embodied linguistically, which is problematic when most of the internet’s knowledge base is written in one language.
For example, while you could argue that “an owl is an owl,” western cultures see owls as wise animals, whereas other cultures see them as the harbingers of death and doom – so how do you classify the meaning of an owl?
Even different colors are perceived and valued differently across cultures, and their meaning culturally ambiguous. Here, the ground truth of such matters doesn’t conform to one single objective meaning.
Ultimately, any AI model that treats the internet as a panacea for global knowledge is prone to bias.
We also have to consider that CLIP learned from a vast selection of images produced by top research facilities.
While we can make no assumptions about the diversity of teams creating such datasets or their methods for reducing bias, these datasets are still potentially culturally situated and have homogenous cultural origins. For example, many early image datasets contained a disproportionate number of white men, which has also led to the downfall of recruitment AIs trained on historical hiring data. The lack of diversity in datasets remains a persistent issue.
Bias is all-pervasive, and it’s incredibly difficult to divorce from both the human consciousness and the consciousness of the AIs that humans create.
When humans evaluate the bias of an AI system like CLIP, it might seem like a harmless academic exercise.
However, if such AIs are deployed into real-world scenarios, the stakes are very high indeed. For example, in 2020, a third black man (known to the media at the time) was wrongfully arrested due to a bad facial recognition match.
There are near-limitless examples of AIs perpetuating bias across healthcare and the criminal justice system, worsening the outcomes of marginalized individuals.
Fast forward to 2022 and it’s concerning that even the most powerful AIs embody bias, often with disastrous consequences.
Defeating AI Bias
AIs that learn from public data run the risk of embodying the bias of that public data. Just because public data is numerous and free doesn’t make it the perfect solution for training advanced AI models at scale.
Carissa Véliz at the University of Oxford highlights two challenges for solving bias – vetting datasets robustly, using human judgment to actively reduce bias – and applying filters to audit datasets for bias prior to training models.
While building datasets from scratch is the most robust way to tackle bias, this is time-consuming, which is what AIs like CLIP are trying to solve.
The truth of the matter is, the most effective solution for tackling bias is ensuring that every instance of AI intended for real-world scenarios is vetted, checked, and tested prior to deployment, and throughout use.
Any and all involvement of human teams is a benefit, not a hindrance, to reducing bias in AI – especially now we’re growing increasingly aware of the consequences.