The Sumerians inhabited Mesopotamia some 6000 years ago, the region between the Tigris and Euphrates rivers, in modern-day Iraq, Kuwait, Turkey, and Syria.
Mesopotamia marks humanity’s transition from small agricultural settlements to larger urban societies. The first cities were built here, including Uruk, which had a complex canal, irrigation system and an administrative hub for governing the local region.
The Sumerians were remarkably intelligent – they’re the reason why we have a sexagesimal counting system for counting time, i.e., 60 seconds in a minute and 360 degrees in a circle.
Among Sumeria’s extraordinary accomplishments is their writing system, which goes by the modern name of cuneiform. Cuneiform involves pressing a reed into clay to form logo-syllabic text. This ancient language was initially used for administrative purposes only, e.g., tracking the movement of slaves or animal transactions.
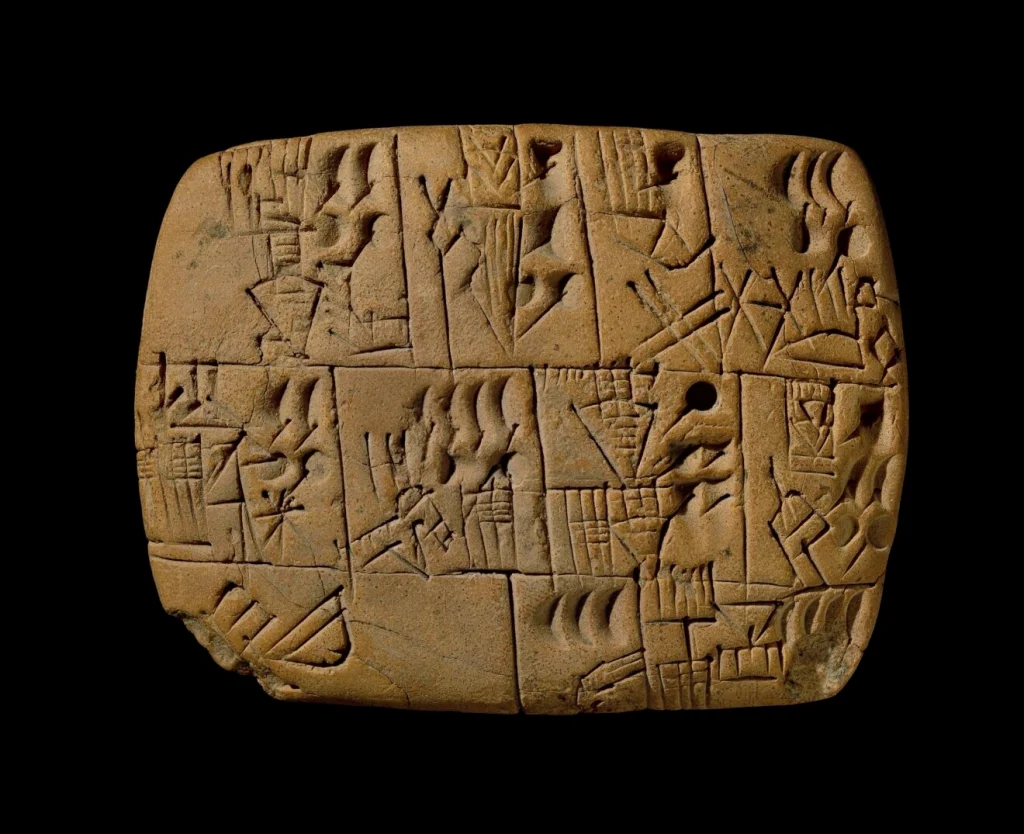
Cuneiform was used for chiefly administrative purposes until around 2700 BC, when poetic and abstract works started surfacing. One of the most famous authors is Enheduanna, a priestess and poet, who wrote the myth of Inanna and Ebih, which describes a battle between a goddess and a mountain.
Perhaps the most famous Sumerian text of all is the Epic of Gilgamesh, which articulates a king’s quest for eternal life, and appears to be the precursor to the Biblical story of The Flood. This 12-tablet work is one of the earliest examples of human literature.
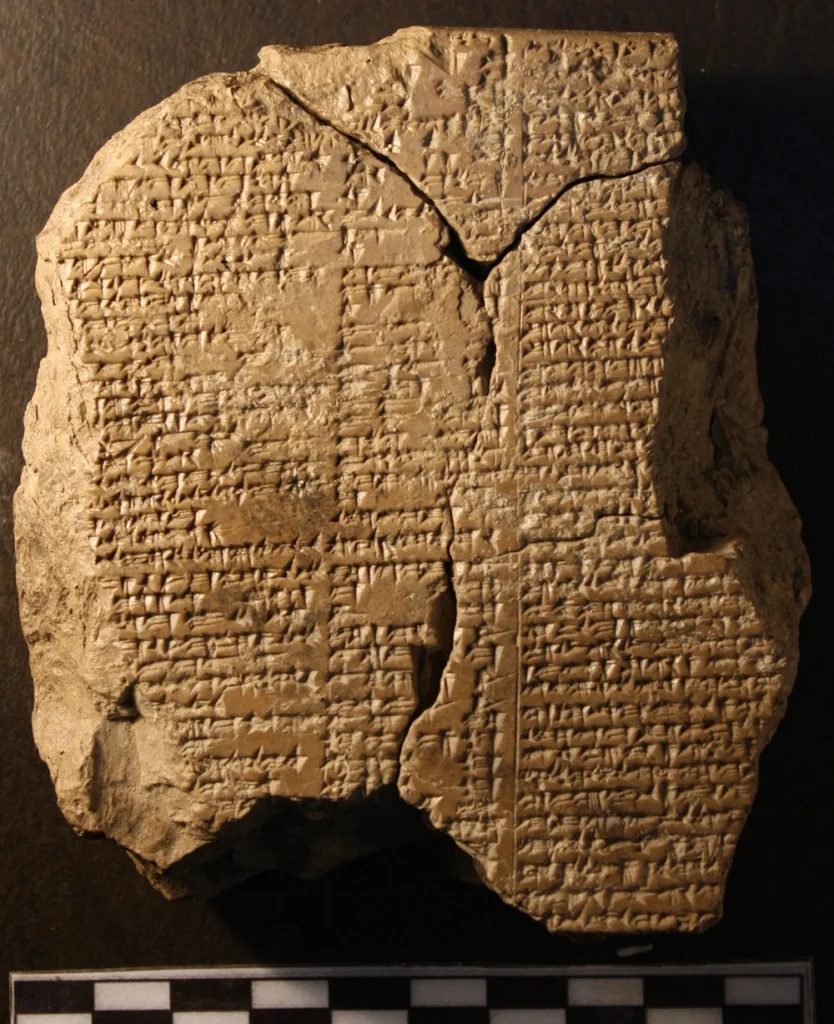
Why is Cuneiform Important?
Cuneiform isn’t a language but a writing system. For example, English and many other Latin languages are written in the Modern Latin Alphabet. If you rearrange the letters in this article and add accents, you could create many Latin-derived or Romance languages.
Cuneiform is the writing system behind around 15 languages that span over 3000 years, including Sumerian, Akkadian, Babylonian, Assyrian, Elamite, Hittite, Urartian, and Old Persian.
To reliably decipher cuneiform is to interpret a vast proportion of humanity’s written history.
“People say the first half of human history is only recorded in these cuneiform tablets,” says Enrique Jiménez at Ludwig Maximilians University in Munich, Germany.
The issue is, only a handful of experts can read and decipher cuneiform, and translating it into modern languages is extremely difficult and incredibly time-consuming. The New Scientist cites that just 75 people can read cuneiform fluently. However, there are some 13,000 tablets in the British Museum and many more in Iraq, and most have probably not been touched, let alone translated.
AI is transforming the field of ancient history by providing researchers with innovative tools to analyze AI ancient texts. These tools open the door to uncovering hidden stories and insights from the past, enabling scholars to decode, interpret and preserve historical wisdom with unmatched efficiency. Through AI, ancient texts are not only safeguarded but also brought to life in ways that were once beyond imagination.
How AI is Helping Decipher Ancient Texts
Enrique Jiménez at Ludwig Maximilian University in Munich, Germany, founded the Electronic Babylonian Literature project to bring together archaeologists, data scientists, and ancient historians to rechart ancient human history.
Jiménez first worked alone but was joined by data scientists and machine learning practitioners who developed algorithms that could piece different parts of the tablets together.
This is fundamental to creating a coherent understanding – one missing piece can completely derail efforts to translate ancient documents. Finding which piece matches which is the first part of the puzzle.
To accomplish this, Jiménez and his team used a machine learning algorithm initially developed to compare different gene sequences. The AI is trained on already-translated components of the text and then predicts what is likely to appear in missing sections. It can also predict which piece or fragment of text belongs with which.
This technique enabled the team to discover missing pieces from the Epic of Gilgamesh. They also found a new Mesopotamian genre – parodies and jokes used to teach children. The team is now piecing together a genre dubbed ‘a hymn to a city,’ which details various parts of ancient life, including temple worshipping and cultic prostitution.
Other discoveries include administrative texts that detail ancient transactions, which also reveal a complex labor landscape where women had access to a variety of important job roles.
However, these techniques use the already-translated form of cuneiform to make their predictions. What if we could use computer vision to decipher ancient texts by simply pointing a camera at them, without pre-translation?
Deepscribe
Researchers trained a machine learning system called Deepscribe on 6,000 hand-annotated images from the Persepolis Fortification Archive, which identify some 100,000 signs.
These texts, written in the Elamite language from around 500 BC, were found in a fortification wall. The researchers understand the text well, helping them create an accurate dataset to train predictive models.
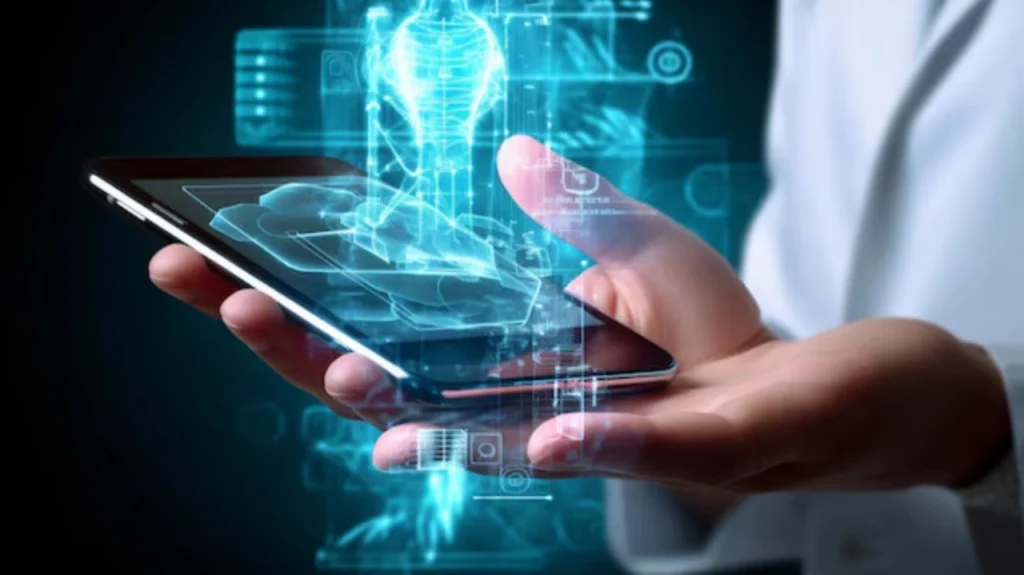
That predictive model aims to help researchers transcribe the text at scale. This speeds up the process of documenting ancient history. The model achieved around 80% accuracy and greatly accelerated the researcher’s translation efforts.
The ultimate goal is to create a portable translation device that can be pointed at various ancient texts for instant translation.
This would help archaeologists identify and analyze ancient texts worldwide without tedious manual translation. Here, AI does much of the heavy lifting, making life easier for humans without ‘taking their jobs’ – a common criticism of AI.
Ithaca
Deepmind was also trained with a similar problem to decipher damaged Ancient Greek tablets at scale. The model helped historians restore the texts with 72% accuracy and could predict the date they were written within 30 years of their actual age. It could even predict the region where the texts were written with 71% accuracy.
The model, dubbed Ithaca, was trained using around 60,000 ancient Greek texts from across the Mediterranean written between 700 BC and AD 500. Inscriptions were labeled with metadata relating to the place and time of writing across a total of 84 ancient regions.
Modern Challenges For Computer Vision and NLP
Using machine learning to read and translate ancient texts is an excellent application of AI technology. Aya Data understands what it takes to create unique AIs that assist those in various professional disciplines, which you can read about in our Case Studies.
Prof. Sanjay Krishnan of the Department of Computer Science at a Neubauer Collegium told Phys.org; “It’s a good machine learning problem, because the accuracy is objective here, we have a labeled training set and we understand the script pretty well and that helps us. It’s not a completely unknown problem.”
Here, data labeling and data annotation transform ancient texts into training data. Krishnan speaks of the objectivity imparted onto the dataset during labeling, which is exceptionally important when dealing with localized, rare, specialist, or domain-specific knowledge (in this case, knowledge of the ancient texts being labeled).
This is analogous to one of Aya Data’s labeling projects which involved labeling maize diseases to create a computer vision AI that could predict the disease a crop was affected by. Here, we used agronomic experts to help us label the data.
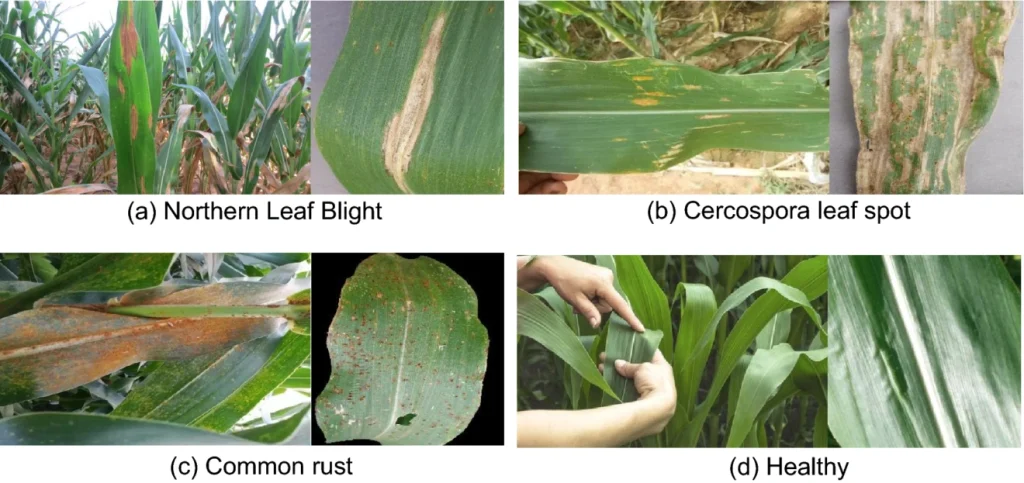
The agronomic experts possessed the localized, specialist or domain-specific knowledge required to create an objective dataset. We translated that knowledge onto the dataset via the data labeling process. The eventual model predicts common maize diseases with 95% accuracy.
Another example is labeling medical images, which again requires a high degree of domain knowledge to ensure that labels genuinely represent the diseases, lesions and other features of the images.
When training models for these types of domain-specific uses, it’s crucial to build accurate datasets that represent the ground truth. This requires a collaboration of data labeling expertise and domain-specific knowledge.