The world is changing at an unprecedented rate, the reality being that we are running out of time to prevent catastrophic and irreversible damage to the climate and environment. World leaders have a global mandate to catalyse real change at the highest level, which is where next-generation technologies such as geoAI can help.
Geospatial AI, or geoAI, is the combination of geospatial science with AI.
Geographic Information Systems (GIS) provide a tremendous resource of planet-wide geospatial data, data that AI can work with at a scale unobtainable via manual handling and processing.
There are several highly-developed GIS platforms available throughout the world, such as SAGA GIS, ArcGIS, Mapinfo Professional, GeoMedia, GRASS GIS and the recent Google Earth Engine, and many of these platforms now integrate AI and ML tools for making use of their spatial data.
Combined with real-time monitoring systems, AI is revolutionising the way we work with geospatial data.
Combining AI and Geospatial Data
The modern GIS is not the static map of old, but rather a living, breathing combination of historical and real-time spatial data that covers everything from weather and climate monitoring to urban development analysis and ecosystem damage following extreme weather events
Geospatial data is used to analyse and evaluate risks from adverse weather conditions, wildfires, drought, flooding and other climate hazards. It can be used to track rainfall and water availability, forestation, soil health and air pollution.
AI is being combined with these ‘living maps’ to utilise their geospatial data in a finer, more granular way than ever before.
For example, ArcGIS showcases examples of their GIS being used to train algorithms that can:
- Detect graffiti, overgrowth or blight hotspots in urban areas
- Automate the data labelling and extraction of road assets, like signs and road markings
- Predict crash risks across road routes
- Detect road cracks and estimate their size
- Extract advanced features from buildings, e.g. their roof type
- Tracking urban trees and foliage
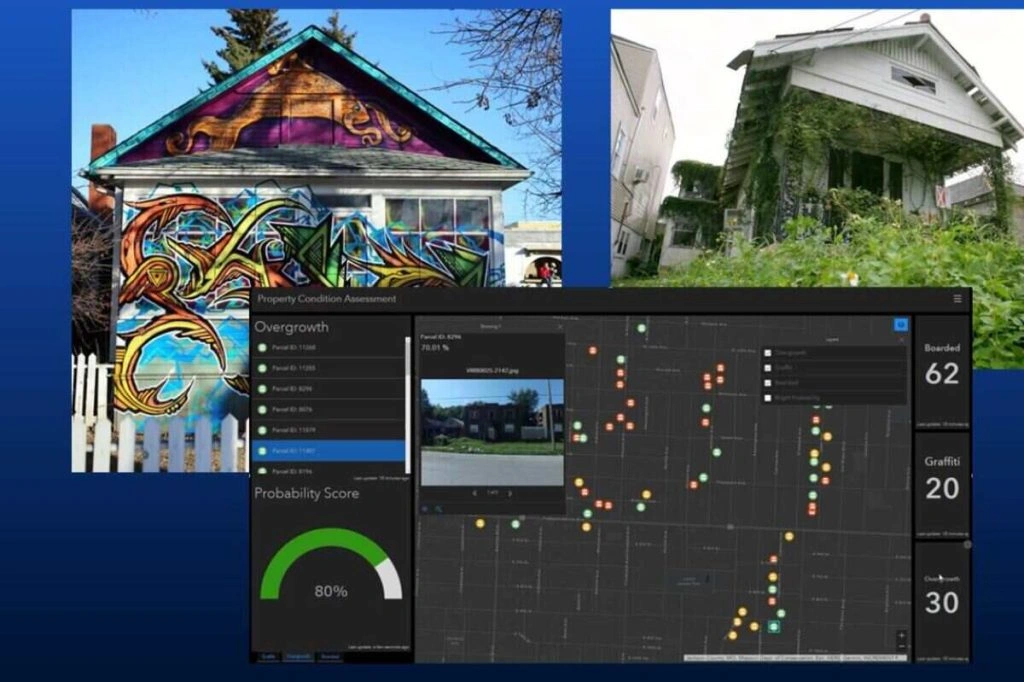
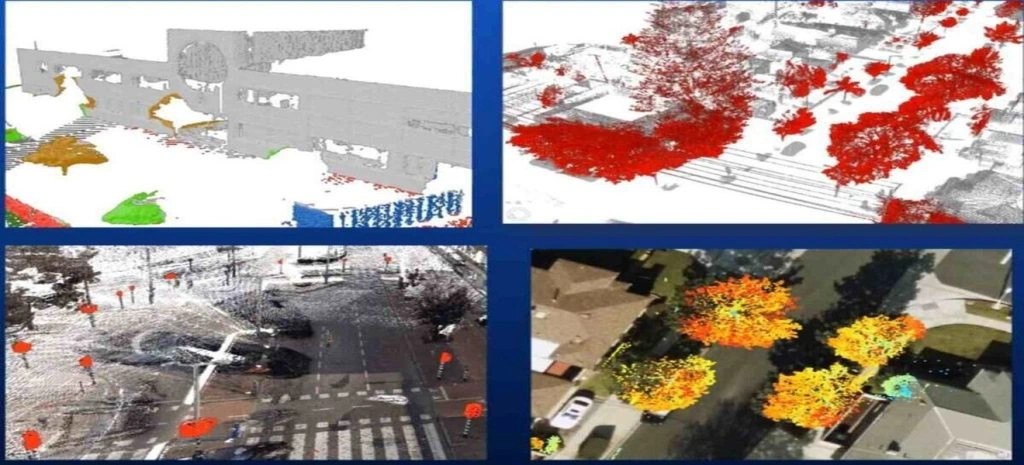
Most of these tasks are achieved through a combination of computer vision and object detection, using data to feed into predictive algorithms. Image labelling and annotation features within the GIS allow deep learning algorithms to go about auto-recognising objects and their characteristics from geospatial images.
The quality of results always depends on the quality of pre-training data labelling. Through a combination of bounding box, semantic segmentation and polygon annotation, virtually any geospatial object can be converted into accurate training data.
Use Case: Geospatial AI and Deforestation
To understand deforestation, we first need an accurate model of the world’s trees to track and analyse. AI is vastly improving the accuracy and detail of these models, mapping forests down to each and every single tree.
- The World Economic Forum overviews Microsoft’s AI For Earth which is being used to map forests accurately with minimal fieldwork, allowing teams to quickly react to natural and human deforestation events.
- AI is being used to map palm oil and fresh fruit supply chains in Indonesia and Malaysia, allowing governments and stakeholders to better understand the impacts of trade.
- A remote monitoring system in Cambodia allows the Cambodian Ministry of Environment to remotely monitor an area of 6.2 million hectares, helping them react quickly to illegal logging and mining operations.
- The final frontier for combating deforestation, the Amazon, is also being analysed with AI to better understand the forest. The data science company Gramener used convolutional neural networks to predict plant and tree species using some 675,000 images, achieving 85% accuracy.
- The Global Observatory further highlights how AI is being used to develop advanced remote monitoring systems capable of tracking and predicting illegal activities and natural deforestation events in the Amazon.
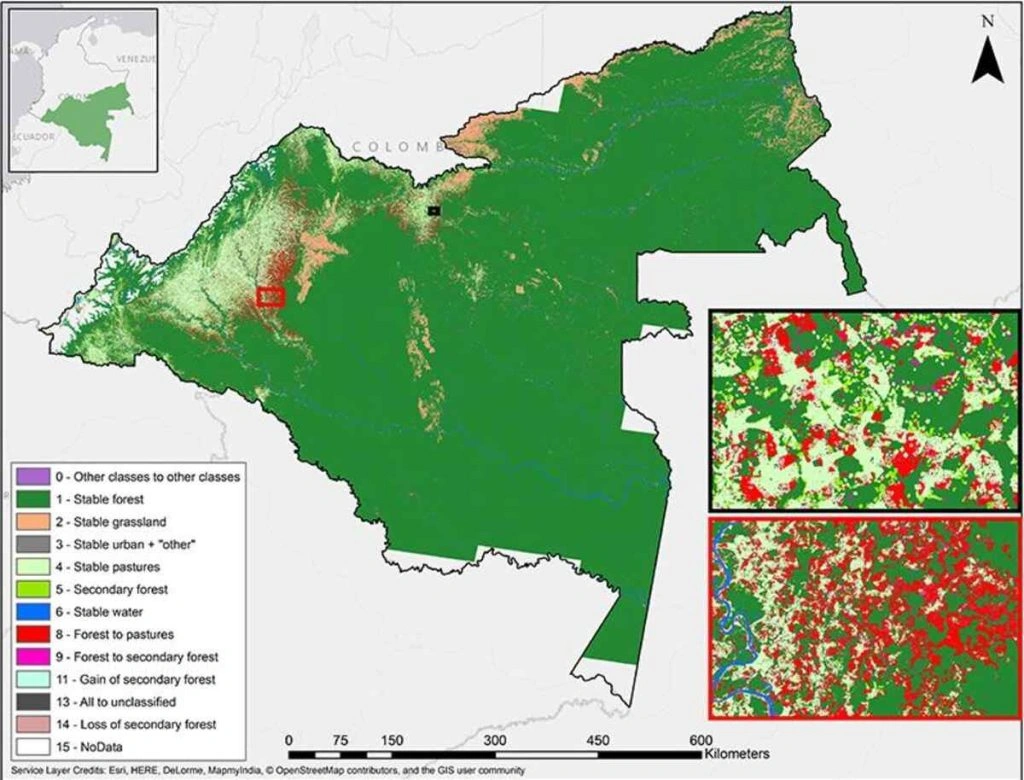
The end goal is producing advanced, self-developing models that accurately predict manmade and natural deforestation events, allowing governments and NGOs to take swift evasive action prior to damage taking place.
Use Case: Mapping Permafrost
Permafrost is one of the greatest climate challenges that we face. Permafrost regions hold some 1,500 billion tonnes of carbon, “that’s about twice as much carbon in the atmosphere, and three times as much carbon than that stored in all the world’s forests”, Dr Sue Natali, an Arctic ecologist tells the BBC.
In collaboration with NVIDIA and the Canadian Space Agency, 3vGeomatics are using AI-assisted tools to map and monitor permafrost across the Canadian Arctic, providing real-time insights into meltage.
Similarly, AI is being used to monitor and predict sea ice loss. IceNet, an AI predictive algorithm, can predict whether sea ice will be present in an area within 2 months with 95% accuracy, which beats traditional physics-based models.
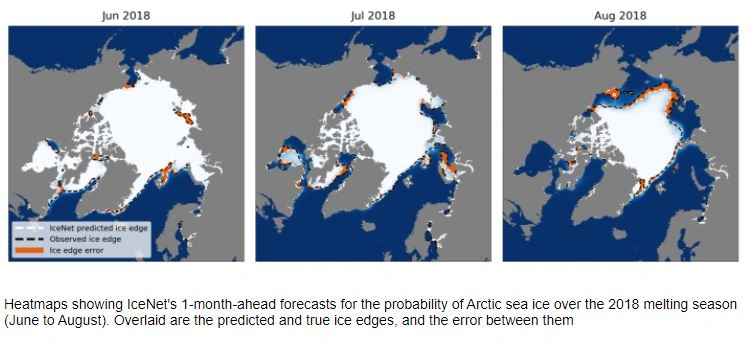
Summary: Using Geospatial AI Technology to Save the Planet
The common themes amongst these examples are speed and accuracy. Speed is always of the essence in time-sensitive systems – shifting to proactive and predictive action from reactive action can help us turn the tables on fast-moving adverse climate and environmental events.
AI is fast and works at scale. Once trained, AI algorithms are able to work with vast volumes of fast-moving data streams, pulling out insights for human teams to investigate further.
As ever, accurate data labelling and data annotation are fundamental in training accurate models. Aya Data are specialists in all forms of data labelling and annotation for AI and ML projects.
Get in touch today to discover how we can help with your data project.