In June 2022, a cross-discipline team of researchers at the ”University of Chicago created an AI” model that could predict the location and rate of crime in the city with 90% accuracy.
The team utilized citywide crime open data between the years of 2014 and 2016, dividing the city into squares of around 1000ft (300m). The resulting model could predict the square where crime was most likely to occur 1-week in advance.
The study itself aimed to analyze the existence of spatial and socioeconomic bias and discovered that crimes in wealthier neighborhoods received more attention and resources than those in lower socioeconomic areas. Researchers also trained the model on data from seven other US cities with similar performance. The data used in the study is publicly accessible, and the algorithms are available on GitHub:
- Opendata.atlantapd.org
- Data.austintexas.gov
- Data.detroitmi.gov
- Data.lacity.org
- Opendata.philly.org
- Data.sfgov.org
- Data.cityofchicago.org
Chicago has a controversial history of experimentation with AI and algorithmic policing. For example, the city produces a gun crime heat map that displays ‘hot spots’ of crime, leading to a famous case of a man being approached by police in his own home due to inferred connections with potential gun crime.
The man, named Robert McDaniels, lived in the Austin district of Chicago, where 90% of all gun crimes in Chicago occur. He had no violent criminal record, just marijuana and street gambling offenses.
Once his name was on the potential violent criminal list, he became isolated and was twice targeted by gun violence. He argues that the incidents were directly or indirectly caused by him being unfairly highlighted as a potential gun criminal.
Once police released details of Chicago’s predictive criminal list, it was revealed that some 56% of black men in the city aged between 20 and 29 appeared on it.
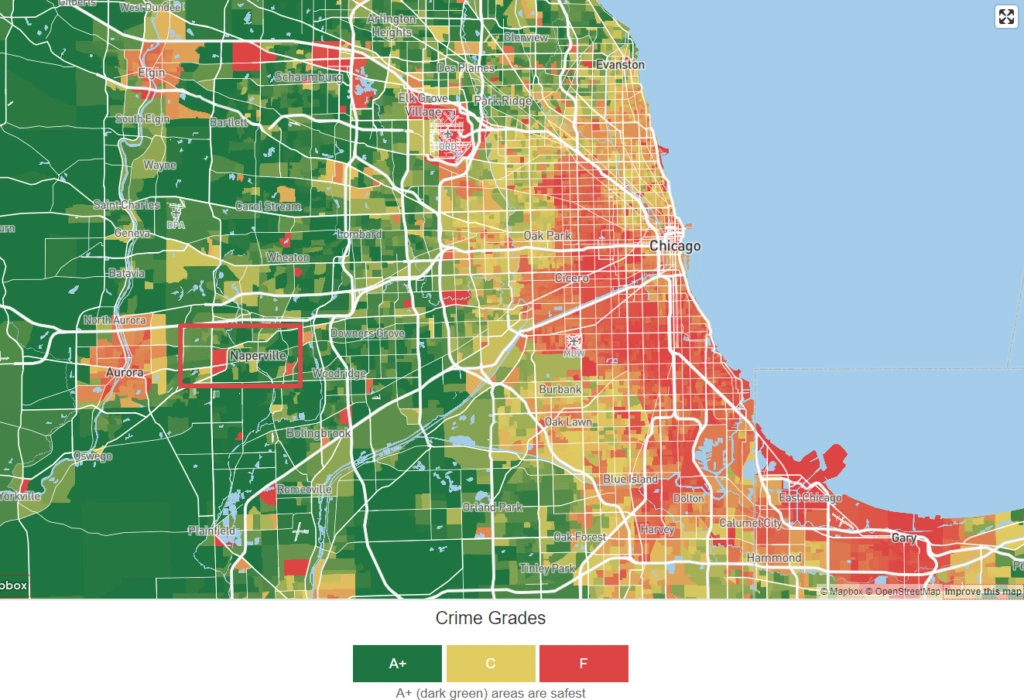
Other predictive policing projects in the USA have led to innocent individuals being approached and harassed by police for petty civil infractions, such as having an unkempt lawn:
“They swarm homes in the middle of the night, waking families and embarrassing people in front of their neighbors. They write tickets for missing mailbox numbers and overgrown grass, saddling residents with court dates and fines. They come again and again, making arrests for any reason they can” – Resident in response to Florida Pasco County’s predictive policing program.
To date, it’s difficult to reconcile the benefits of predictive policing with its proven negative impacts.
Algorithmic policing has a reputation for exacerbating bias rather than reducing it, whereas AI should reduce bias if trained and utilized correctly. It boils down to the quality of datasets and how human decision-making intersects with AI.
AI’s Relationship with Human Decision-Making
AI is often seen as incorruptible. With its superior, objective treatment and omniscience of data that the human brain cannot perceive itself, surely AI holds the right answers to complex questions?
In these situations, AI demonstrates how dangerous it can be when used irresponsibly. AI is as corruptible as the humans that use it – and algorithms don’t always remain in the hands of those intended.
The head researcher of the latest attempt to predict crime in Chicago, Ishanu Chattopadhyay, was keen to point out that the model only predicts the area, not the suspect; “It’s not Minority Report,” he told New Scientist. Indeed, the intent beyond this particular study is to highlight potential bias – and not reinforce it.
But the key question is, what about the data used to train such models? The data itself is subject to bias, thus biasing the eventual model. In other words, the ground truth is somewhat masked by bias that infects the objectivity of the datasets.
Chattopadhyay responded to these potential issues, highlighting that they excluded citizen-reported crimes involving petty drug crimes and traffic stops and concentrated on violent and property crimes that were more likely to be reported. Nevertheless, biased data is sure to produce a biased model – which is something data labelers and humans-in-the-loop can help prevent.
Digital Twins for Policing and Crime
Digital twins are digitized models of systems that enable us to experiment with a system in a non-destructive environment. Data is taken from the real world and impregnated into a predictive model – a ‘digital twin’ of the real world.
The concept of digital twins dates back to NASA’s space programs in the 70s, most notably Apollo 13. In 1970, Apollo 13 suffered an explosion in an elliptical orbit around 200,000 miles from Earth.
NASA was already using simulators to predict their systems in space, enabling them to simulate space physics and environments from their base on Earth. So when Apollo 13 exploded, NASA’s team simulated possible sequences that would safely get the crew back to Earth.
By simulating these sequences in their primitive ‘digital’ twin environment, NASA could safely execute a sequence that sent the astronauts back towards earth. On April 17th, 1970, Apollo 13 splashed into the Pacific Ocean.
We’re now seeing the application of digital twin modeling on crime and other social phenomena. Chattopadhyay said, “We created a digital twin of urban environments,” “If you feed it data from what happened in the past, it will tell you what’s going to happen in the future”.
The smart cities of the future will probably be hosted as digital twins – models that public and private sector actors can interact with to model everything from crime to traffic and energy consumption.
How humans will learn to interact with these complex models while navigating their shortcomings is a work in progress.
Interested in navigating similar challenges and finding AI-powered solutions? Contact us today to speak to an expert.