Data labeling is a fundamental step in building effective machine learning and AI models. It involves the structured process of tagging raw data-such as images, text, audio, or video with meaningful labels or annotations. These labels act as training signals, enabling AI algorithms to learn how to identify patterns, classify objects, and make accurate predictions. Without high-quality data labeling, even the most advanced AI systems struggle to perform reliably.
In this comprehensive guide, we will explore the intricacies of data labeling, its significance, methods, best practices, and more. Join us on this journey to unlock the power of data labeling in the world of AI.
What is Data Labeling?
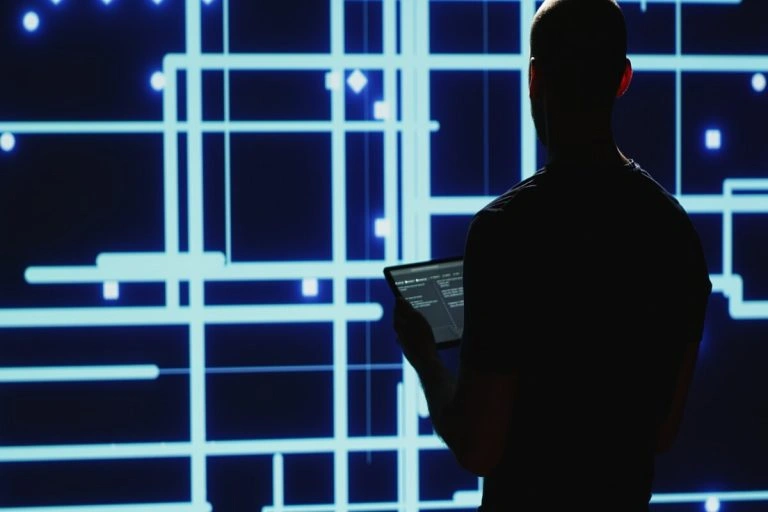
Data labeling, also known as data annotation, is the process of assigning meaningful tags or metadata to raw data. These labels enable machine learning algorithms to interpret and learn from the data accurately, making data labeling a critical foundation for developing reliable and high-performing AI models.
In machine learning, the term “ground truth” refers to the labeled dataset used to train and evaluate a model. The performance of any model is directly tied to the quality of this ground truth. That’s why investing in precise, high-quality data labeling is essential for building accurate and trustworthy AI systems.
Why Use Data Labeling?
Data labeling, a fundamental process in the world of artificial intelligence and machine learning, is like the guiding star that leads these technologies towards effectiveness and accurate predictions. Here’s why harnessing the power of data labeling is essential:
- Precision Amidst Complexity: In a digital world filled with vast and complex datasets, data labeling acts as the compass that helps AI algorithms navigate and understand the intricacies. It’s akin to providing a roadmap for these algorithms to identify specific landmarks within the data.
- Fueling the Learning Engine: At the heart of machine learning lies the concept of supervised learning. Data labeling is the key that unlocks this learning approach. By labeling data, you’re essentially giving AI models the examples they need to grasp concepts and make accurate predictions.
- Contextual Comprehension: Think of data labeling as adding captions to images. Just as captions provide context and meaning to a photograph, labels breathe life into raw data. They enable AI to not just see information but understand it in context.
- Custom Tailoring: Data labeling allows you to tailor AI models to suit your specific needs. Whether you’re teaching a model to identify dog breeds in images or sentiments in text, the labels provide the guidance required for specialization.
- Real-World Applications: From autonomous vehicles interpreting road signs to chatbots understanding customer inquiries, data labeling fuels AI applications in the real world. It’s what empowers these systems to interact with humans and their surroundings effectively.
- Continuous Improvement: AI models are like eager students, always ready to learn. Data labeling doesn’t stop at initial training; it’s an ongoing process of refinement. Regular updates and feedback loops ensure that AI keeps getting better over time.
- Trust and Transparency: In an age where AI impacts critical decision-making, transparency is paramount. Data labeling helps in making AI models more interpretable. Understanding why a model made a particular decision builds trust and facilitates accountability.
- Compliance and Ethics: In industries like healthcare and finance, compliance with regulations and ethical standards is non-negotiable. Data labeling ensures that AI systems handle sensitive information responsibly and in line with legal requirements.
- Multi-Domain Adaptation: Data often comes from various sources and domains. Data labeling makes it possible for AI models to adapt and thrive in diverse environments, making them more versatile and adaptable.
- Realizing AI Potential: Ultimately, data labeling is the catalyst that unlocks the full potential of AI. It transforms machines from mere calculators into intelligent decision-makers, capable of handling complex tasks and making sense of the data-rich world we live in.
In essence, data labeling is the art and science of injecting understanding and intelligence into AI. It’s the reason why AI can see, read, and interpret our world, making it an indispensable tool for solving problems and driving innovation in countless fields.
How Does Data Labeling Work?
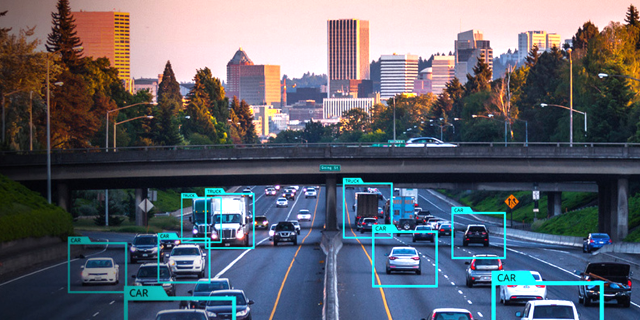
Data labeling is a bit like teaching a child to recognize objects by pointing them out and providing names. It involves these key steps:
- Data Collection: First, you gather the data you need, whether it’s images, text, or any kind of information.
- Data Tagging: Then, you meticulously add labels or tags to this data, like saying, “This is a cat,” or “This sentence is positive.” These labels help AI understand what it’s looking at.
- Quality Assurance: Just as you’d double-check a child’s learning, data labeling includes quality checks. Experts review the labeled data to ensure accuracy and consistency.
- Model Training: Now, it’s time to teach the AI. You feed it this labeled data, allowing the AI to learn and make sense of the patterns in the labels.
- AI Application: Finally, the AI uses what it’s learned to make predictions or classifications. It’s like the child recognizing a cat or understanding a positive statement without your help.
In a nutshell, “data labeling” is the process of adding context to raw data by assigning labels, allowing AI systems to interpret, learn from, and make informed decisions based on that data.
Data Collection
Collecting diverse and specific data is the foundation of data labeling. The quality and variety of data collected directly impact the effectiveness of AI models.
Data Tagging
Data tagging involves human labelers labeling the collected data with relevant information. For example, in image annotation, objects within images are tagged with labels that describe them.
Quality Assurance
Ensuring the accuracy and consistency of labeled data is critical. Quality assurance processes involve checks and reviews to eliminate errors.
Model Training
Labeled data is used to train machine learning models. These models learn to make predictions or classifications based on the labeled examples.
Common Types of Data Labeling
write a long section about the common types of data labeling using this paragraph as a starting point:
The practice of data labeling assumes diverse forms, including image annotation, text classification, sentiment analysis, and beyond. The choice of labeling methodology hinges on the specific nature of the task and the characteristics of the dataset under consideration.
Image Annotation
The process of image annotation involves tagging visual data with relevant labels to provide context to an image. For example, an image of a landscape might be annotated with labels such as “trees,” “mountains,” and “river.”
The level of detail in image annotation can vary greatly, from simple labels that describe the scene broadly to complex labels that specify individual objects and their positioning. Image annotation is an essential component of computer vision, an area of artificial intelligence (AI) that aims to train computers to interpret and understand visual data.
Applications of computer vision range from autonomous vehicles that need to recognize road signs and obstacles, to medical imaging technology that can identify anomalies in scans.
Text Classification
Text classification, also known as text categorization, is another of data labeling. This process involves assigning predefined categories to unstructured text data. For instance, a dataset of news articles might be classified into categories such as “politics,” “sports,” “business,” and “entertainment.” Text classification is a key element in many natural language processing (NLP) tasks, including spam detection, sentiment analysis, and document tagging. It allows machines to understand and organize vast amounts of textual data, making it a crucial foundation for many AI applications.
Sentiment Analysis
Sentiment analysis, often associated with text classification, involves labeling text data based on the sentiment expressed within it. This could range from simple classifications such as positive, negative, or neutral, to more nuanced labels that capture specific emotions like anger, joy, or sadness.
Sentiment analysis is often used in social media monitoring, customer feedback analysis, and market research, allowing businesses to gauge public opinion and customer sentiment towards their products or services. It’s a powerful tool for understanding human emotions and opinions at scale.
Semantic Annotation
Semantic annotation involves annotating data with metadata that provides more context and meaning. In semantic annotation, labels aren’t just tags; they’re meaningful descriptions that enable a deeper understanding of the data.
For instance, in a dataset of scientific articles, semantic annotation could involve tagging each article with its research field, the methodologies used, the results obtained, and other relevant information. This type of annotation is particularly beneficial in fields like semantic search and knowledge graph construction, aiding in information retrieval and data linking.
Video Annotation
Video annotation is similar to image annotation but involves labeling sequences of images or videos. This can include object tracking, action recognition, or scene understanding. Video annotation is a complex process due to the temporal dimension added by the moving images. It’s a component of many AI applications, including surveillance systems, autonomous vehicles, and sports analytics.
What Are Some of the Best Practices for Data Labeling?
Ensuring the efficacy of data labeling demands the adherence to a set of best practices:
- Collect Diverse Data: Enrich your dataset with diverse examples to bolster model generalization.
- Establish Clear Annotation Guidelines: Crafting meticulous and comprehensible annotation guidelines for your labeling teams ensures consistency throughout the labeling process.
- Robust Quality Assurance: Institute a robust quality assurance framework to pinpoint and rectify errors in labeled data.
- Optimal Annotation Pipeline: Select the most fitting annotation pipeline for your project’s unique requirements.
- Open Communication: Foster transparent communication channels between data labelers and project stakeholders.
- Continuous Feedback: Provide regular feedback to data annotators to enhance their performance and ensure ongoing refinement.
- Pilot Projects: Run pilot projects to fine-tune your labeling approach and identify areas for improvement.
- Inclusive Tools: Employ inclusive tools and integrated management systems for maximum efficiency.
What Should I Look for When Choosing a Data Labeling Platform?
Selecting a data labeling platform warrants careful consideration of several critical factors:
- Quality Assurance Processes: Verify the platform’s quality assurance processes to ensure the accuracy and reliability of labeled data.
- Guaranteed Privacy and Security: Ensure the platform adheres to stringent privacy and security standards to safeguard your sensitive data.
- Technical Support and Documentation: Assess the availability of robust technical support and comprehensive documentation to assist with any challenges or queries.
Key Takeaways
Data labeling is a critical stage in building accurate and reliable AI models. This detailed process includes data collection, annotation, quality assurance, and integration into model training. By following best practices and choosing the right data labeling platform, you can unlock the full potential of your data and drive your AI initiatives toward measurable success.